Deep Learning at ePotentia
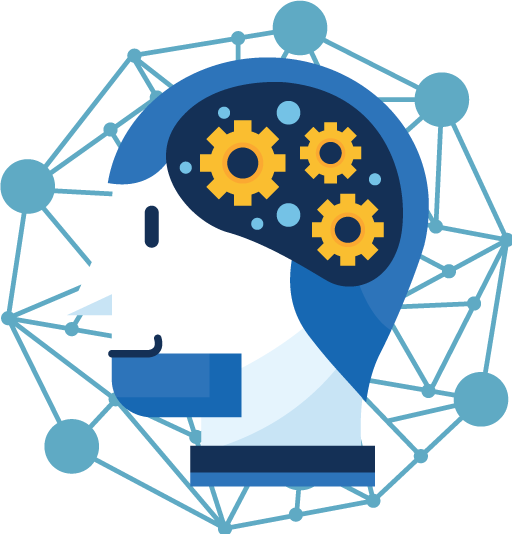
Deep Learning
Deep learning is widely regarded as one of the most innovative and disruptive technologies in the 21st century. It’s impact stems from its ability to extract highly complex patterns from big data through architectures based on the human brain. The data they can handle is much more varied than classical programs and statistical methods, ranging from image data to graphs and text. Of course the size and quality of the data set will be strongly linked to the performance, but modern techniques such as data augmentation and transfer learning can allow us to create models even for medium-sized datasets.
Image Analysis
Image analysis remains the prime example of deep learning applications. These models can efficiently encode the information within the image and then use it for various tasks. A classification task is the simplest example. Does this image contain one of 26 letters? Is the product in this image good or bad? The output does not however have to be a class, but could be any form of data. The good and bad product could become a quality score from 1 to 100, creating a regression model. A segmentation model would color the good and bad regions in the image, classifying every pixel. Object detection detects multiple objects within a single image, outlining them with a box that could then be classified or counted. These examples are just the tip of the iceberg. Have an interesting image dataset? Don’t hesitate to contact us!
Text Analysis
Text analysis often referred to as Natural Language Processing (NLP) offers a second big field of application for deep learning. For classical applications capturing the nuances of grammar, spelling and context within text was inherently difficult. Similarly to images, deep learning models are capable of encoding this information and transforming it to an output of score. One common family are so-called seq2seq models, transforming one sequence of text into another. A similar-sized output model is, for instance, a translation model. Transforming the grammar of one language into another. Deep learning can, however, perform many other tasks. Summarizing text is another prime example with text as output. Nothing stops you either from using the text encoding into a regression or classification task. Prime examples are sentiment analysis, where text is scored based on emotion, categorizing text based on subject or providing a quality score for a text segment.
From tabular data to graphs and atoms
One of the biggest advantages of deep learning is its versatility. Architectures are now available for many types of data and are pretty much guaranteed to outperform previous techniques, if you have a lot of data. Tabular or numerical data seen in many applications from sales to research can be easily processed. Special techniques exist for time series analysis, with applications ranging from stock market prediction to music generation. Collaborative filtering uses this type of data to create advanced recommendation systems. Deep learning can even be used to study graphs, which can represent anything from an affiliate network to the atoms in a crystal.
Workshops
Want to learn how to apply modern data techniques in-house? We provide both beginner and advanced workshops tailored to your applications.
Development
Gathering new data? Visualizing it on an app or website? Our developers are ready to assist you in creating and deploying data-based applications..
Consulting
In need of data analysis or just advice? Our consultants can provide you with the necessary answers on statistics, machine learning and data-related IT.